
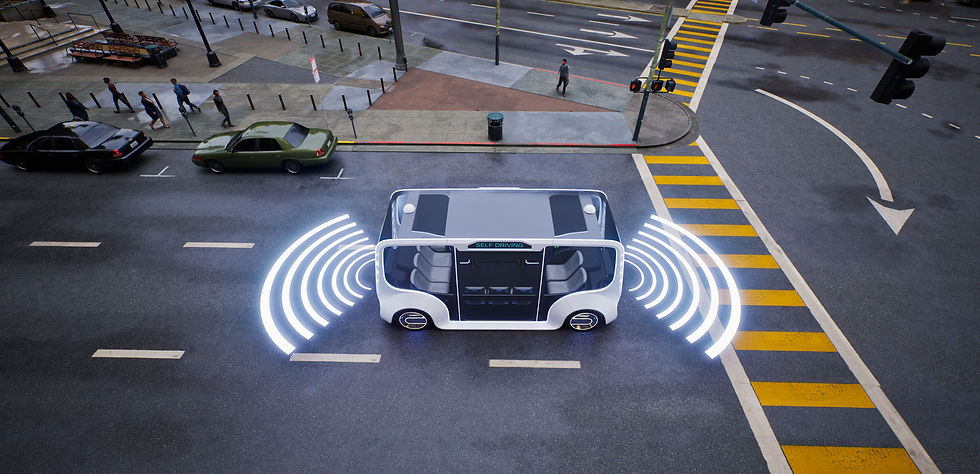
Giuseppe Barile
Mar 31, 2025
From laboratories to reality: Vision-Language-Action models are revolutionising interaction between robots and the physical world
Vision-Language-Action (VLA) models are at the forefront of artificial intelligence applied to robotics, with a significant impact on areas such as industrial automation, autonomous navigation, and healthcare. These multimodal systems combine visual perception, linguistic understanding, and action capabilities, creating robots that interact with the physical world more naturally and efficiently. Research in this field is developing rapidly, opening up new possibilities and challenges to be addressed.
At the heart of VLA models are three core modules that work together to process visual and linguistic information and respond with precise actions. The first module, dedicated to vision , uses advanced neural networks such as CNNs (Convolutional Neural Networks) and ViT (Vision Transformers) models to extract information from images. These are capable of recognizing complex objects, scenes and situations, thanks to the use of pre-training on large datasets. In this context, semantic segmentation techniques are also used to improve spatial understanding and object detection.
The second module is dedicated to natural language . Here, state-of-the-art transformer models such as BERT, T5, GPT-4, and PaLM 2 are used to process and generate coherent linguistic responses. They are trained on large corpora of data and use advanced methods such as cross-attention and few-shot learning to quickly adapt to new contexts, even when the available information is limited. The goal is to ensure that the system can correctly correlate words and images, for understanding language in relation to visual stimuli.
The third module is about action . Advanced decision-making algorithms, such as reinforcement learning (RL), are used to make decisions and perform actions. Thanks to techniques such as Proximal Policy Optimization (PPO) and neural networks for motion planning, they are able to generate optimal action trajectories, dynamically adapting to the situations that arise. The use of imitation learning, which allows the robot to learn by observing the actions of humans, is another key aspect in the evolution of these technologies.
A novel aspect of VLA model research is the use of multimodal fusion . Mechanisms such as cross-modal attention and the use of common embedding spaces allow for the seamless integration of visual and linguistic information, improving the ability to generate coherent responses and precise actions. The most advanced models, such as DeepMind's Flamingo, use generalized attention approaches, capable of efficiently correlating data from different modalities, while techniques such as contrastive learning refine the semantic alignment between vision and language.
The applications of VLA models are wide and varied. In robotics , the ability to understand and interact with the environment in real time makes these models ideal for complex tasks such as object manipulation or autonomous navigation. In particular, projects such as RT-2, which integrate large-scale pre-trained models with robotic control, are able to significantly improve the performance of robotic systems, even in previously unexplored environments. Open-source models such as OpenVLA, with their versatile and highly efficient approach, are also demonstrating how robotics can benefit from VLA systems to improve accuracy and adaptability in real-world settings. However, there are significant challenges to address. One of the main difficulties concerns the adaptability in real-world scenarios, where conditions can vary greatly from the training data. Furthermore, computational efficiency remains a barrier , as these models require considerable resources that limit their use in devices with limited computing power. Advanced semantic understanding, the ability to reason about abstract concepts and complex scenarios, remains an open question, despite advances in linguistic and visual grounding techniques.
The future of VLA models is bright, with ongoing research aiming to make these systems even more versatile and scalable. Approaches such as 3D-VLA, which integrate 3D perception, and improved motion planning, are expanding the capabilities of these models, allowing them to understand and act in complex three-dimensional environments . As more powerful models continue to be developed and practical applications expand, these models could further revolutionize robotics, paving the way for a future where robots not only respond to commands, but can interact and understand the world in ways similar to humans.